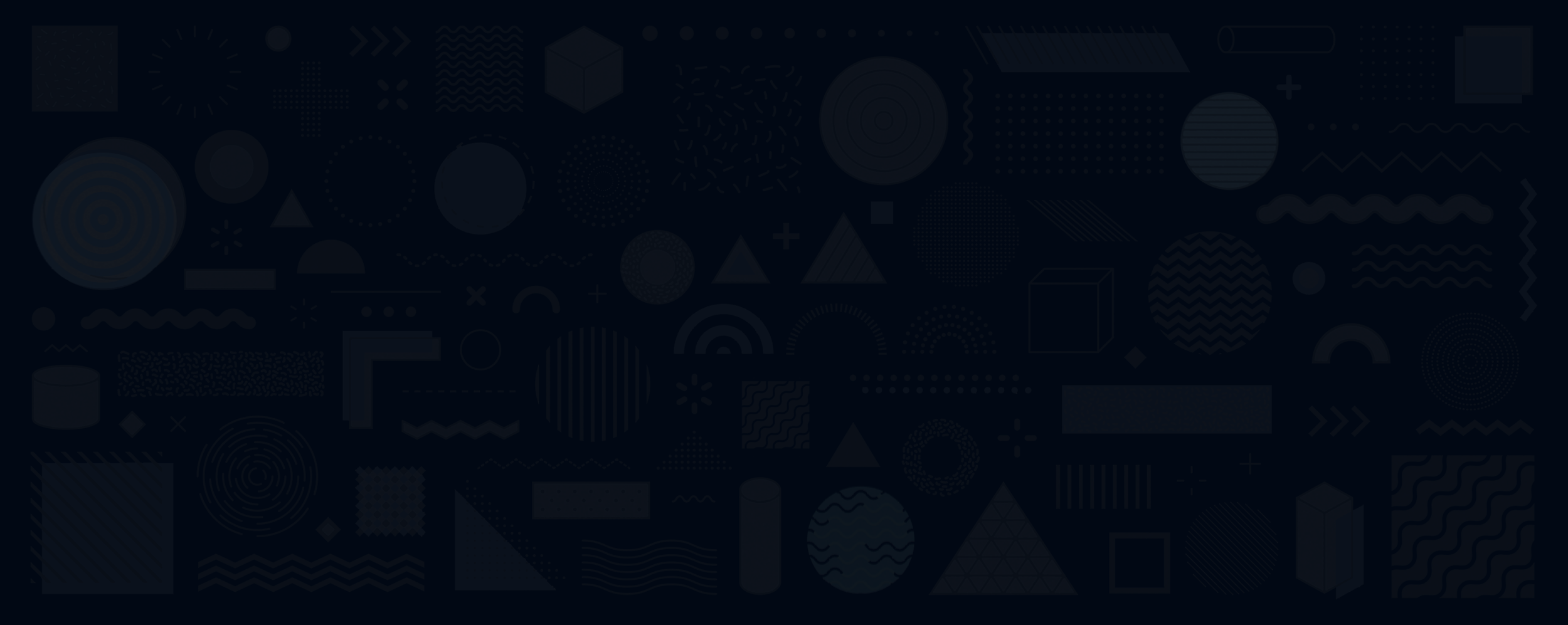
Retrieval-Augmented Generation (RAG)
RAG combines information retrieval with Generative AI, making AI responses more accurate, contextual, and data driven.
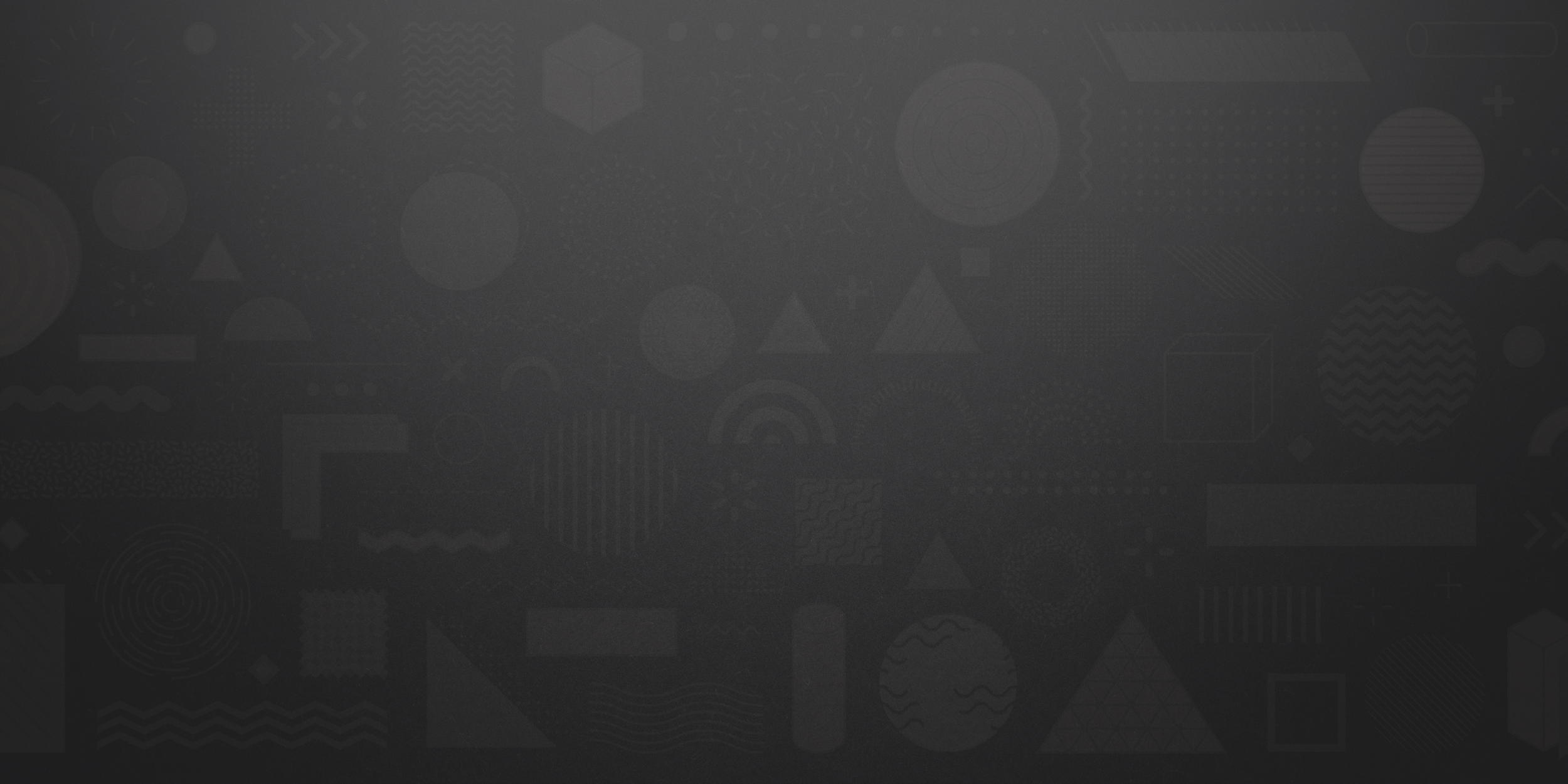
Improved Search Accuracy & Reliability
Grounded in Real Data – Instead of generating responses based on pre-trained knowledge alone, RAG pulls real-time, relevant information from your proprietary databases.
Reduces Hallucinations – Unlike standard Generative AI models that can make up facts, RAG ensures AI-generated content is factually correct by citing retrieved sources.
Enhanced Search & Knowledge Discovery
Context-Aware Answers – Unlike traditional keyword searches, RAG understands the meaning behind a query and retrieves the most relevant documents before generating responses.
Works with Unstructured Data – RAG can extract information from PDFs, CAD files, technical manuals, and engineering documents—even if the data is scattered.
Multi-Modal Retrieval – For visual search applications, RAG can retrieve both text-based and image-based results, making it perfect for engineering, e-commerce, and manufacturing.
Faster Decision-Making & Productivity Boost
Summarizes Large Data Sets – Engineers, designers, and analysts can quickly extract key insights from long documents, manuals, and reports.
Automates Technical Support – RAG can answer complex customer and employee queries by pulling from help center articles, engineering databases, and past support tickets.
Saves Time for Experts – Instead of manually searching through databases, teams get AI-generated, context-rich responses instantly.