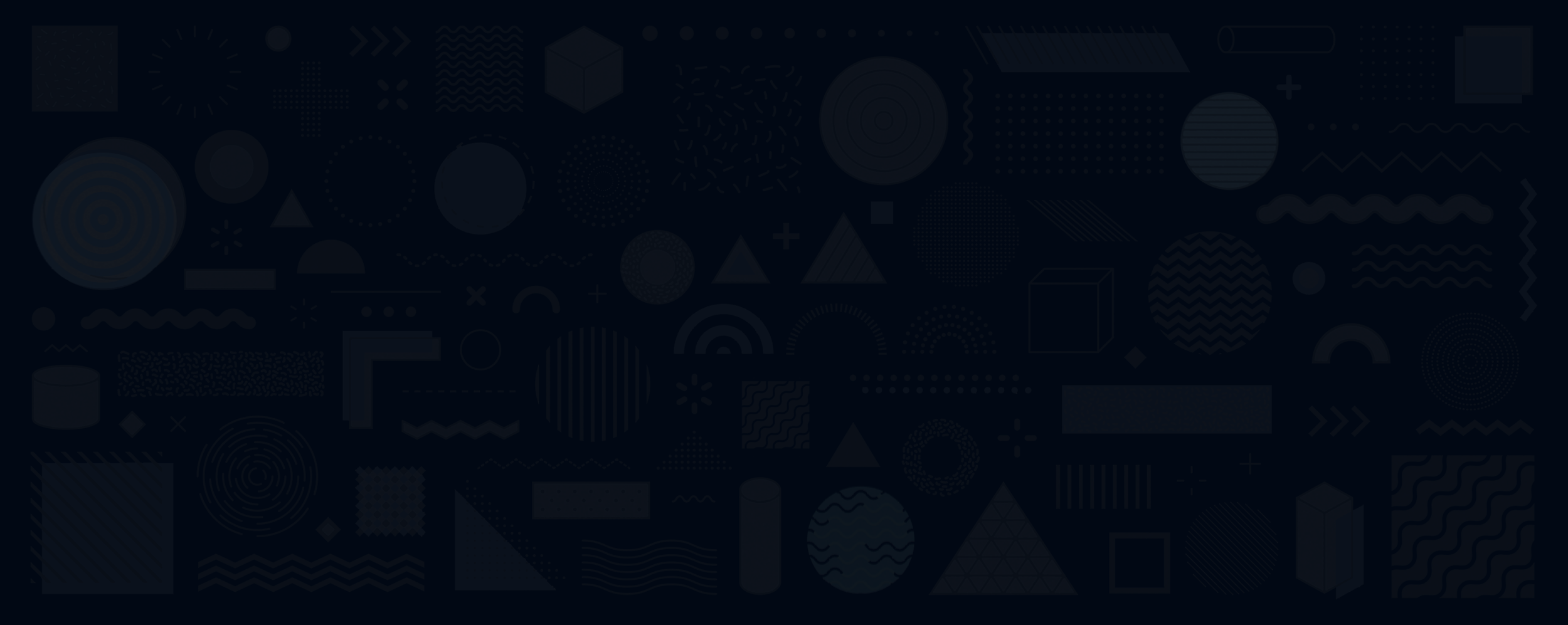
AI-powered Visual Search
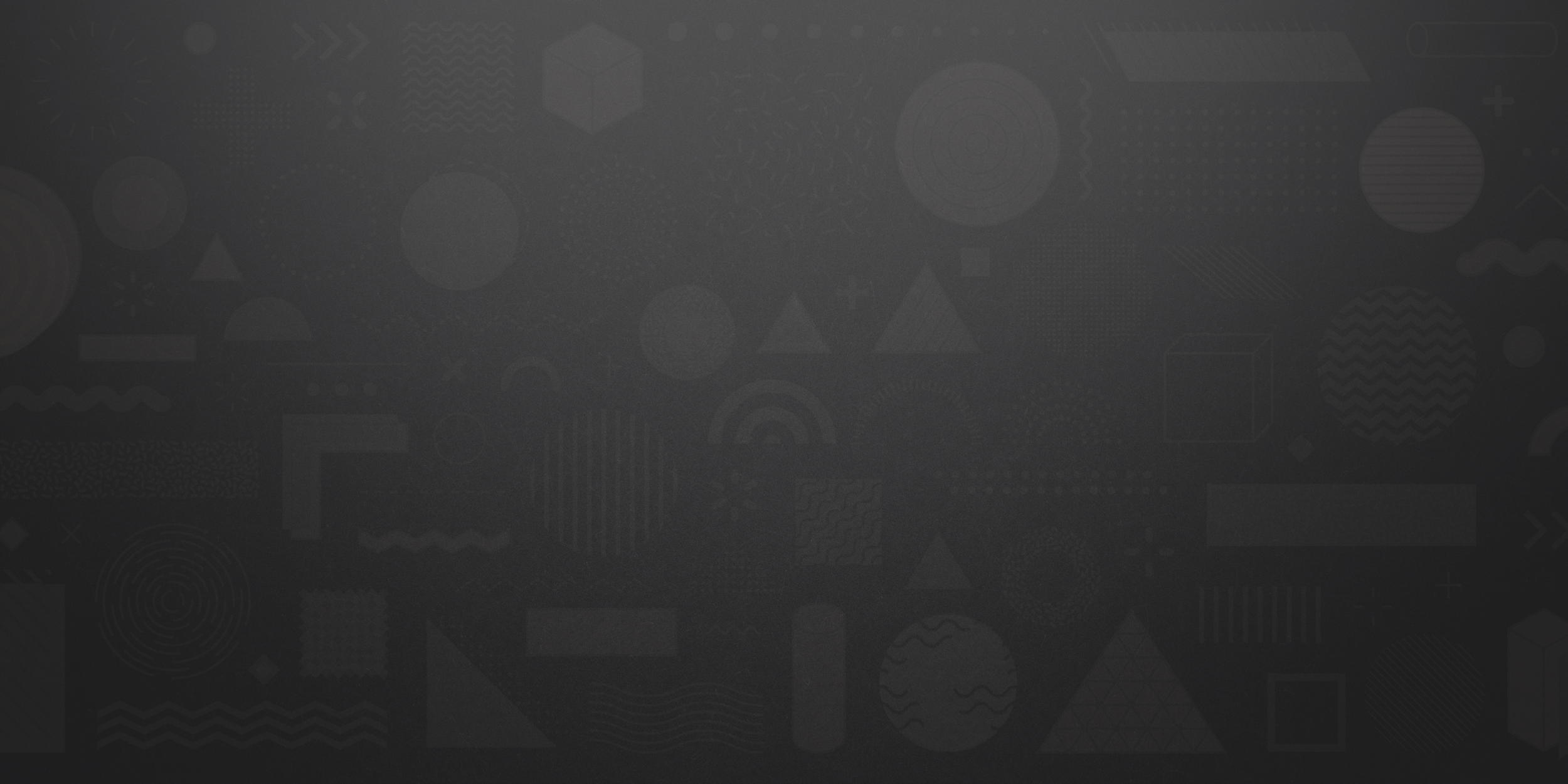
Understanding AI-Powered Visual Search
Computer Vision
Computer vision is the foundation of visual search. It enables machines to analyze and understand visual content by extracting patterns, shapes, colors, textures, and object relationships from images or videos.
Object Recognition – Identifies objects in an image (e.g., a car, a tool, or a machine part).
Feature Extraction – Breaks down images into essential components like edges, colors, and textures.
Semantic Understanding – Interprets relationships between objects in an image (e.g., a person holding a smartphone).
Deep Learning & Neural Networks
AI visual search relies on deep learning, particularly Convolutional Neural Networks (CNNs), which are specialized in processing visual data.
How CNNs Work:
They learn hierarchical features from images (e.g., detecting edges in early layers and complex objects in deeper layers).
The network is trained on millions of labeled images to recognize patterns and similarities.
Once trained, the model can recognize similar images in new searches.
Popular Architectures for Visual Search
ResNet – Helps in deep feature extraction.
VGGNet – Good for object recognition and classification.
EfficientNet – Optimized for fast and accurate image recognition.
Multi-Modal AI (text+image)
Combines visual data with text, audio, or other inputs for richer understanding.
Example: A user searches for “red sneakers” and uploads an image of their favorite shoes. AI refines the search using both visual and textual features to find the closest match.
Technologies used:
CLIP (Contrastive Language–Image Pretraining) by OpenAI
Vision Transformers (ViTs)
Embedding Models
Embedding models convert images, text and audio into dense numerical vectors that encapsulate their features and characteristics. These embeddings enable efficient and scalable rapid retrieval by allowing vector searches to compare and rank results based on similarity within a shared vector space. This approach is particularly effective for large databases.
How Does AI-Powered Visual Search Work?
AI-powered visual search enables machines to analyze, recognize, and retrieve images based on visual content and mimics human vision using advanced computational capabilities.
Step-by-Step Process
Image Input & Preprocessing
A user uploads an image, takes a photo, or selects an existing image from a database.
The system enhances the image by adjusting brightness, contrast, and noise reduction to improve recognition accuracy.
The image is resized and converted into a format suitable for processing.
Feature Extraction with Deep Learning
Enhances training data by creating synthetic images, improving feature extraction, and enabling context-aware analysis. For example, GANs (Generative Adversarial Networks) refine low-quality images for better recognition accuracy.
Image Embedding & Vector Representation
The extracted features are encoded into a vector embedding—a unique numerical representation of the image
The embedding space allows images with similar characteristics to be grouped closely together.
These embeddings are stored in a vector database
Similarity Search & Matching
AI compares the input image’s embedding with stored embeddings in the database.
It uses similarity metrics like:
Cosine Similarity (Angle-Based Similarity) – Think of each image or object as an arrow (vector) in space. Cosine Similarity measures the angle between two arrows. If the angle is small (close to 0 degrees), the two objects are very similar. If the angle is large (close to 90 degrees), they are very different.
Euclidean Distance (Straight-Line Distance) – Imagine plotting two points on a graph. Euclidean Distance measures the straight-line distance between them. If the distance is small, the objects are very similar. If the distance is large, the objects are very different.
Key Difference
Cosine Similarity checks if two things are pointing in the same direction.
Euclidean Distance checks how far apart two things are.
What are some industries that can benefit from AI-Powered Visual Search?
AI-powered visual search is transforming multiple industries by enabling image-based information retrieval, object recognition, and automated classification. Here are some industries and their key applications:
Retail & E-Commerce
Automotive & Aerospace
Healthcare & Medical Imaging
Architecture, Engineering & Construction
Metallography
Agriculture
Purchasing & Procurement
Quoting & Pricing
Education & Research
Logistics & Warehousing
What is AI-Powered Visual Search?
AI-powered visual search allows users to find similar images, objects, or products by analyzing visual content instead of relying on just text, and it revolutionizes the way we find and interact with images. By leveraging computer vision, deep learning, Multi-Modal AI, and Embedding Models, it delivers fast and accurate results across industries.